The Public authority for Civil Information (PACI) passing as responsible to record data about people businesses and addresses all these entities are linked together with a unique identifier called a person number.
How is PACI implemented?
we are using mainframe as our data repository in 2011 passes started the GIS program and we have just enabled our mainframe data with a 99.7% in 2013. PACI started to provide GIS services to government private and start-ups.
Currently, we have more than 100 partners sharing in our DNA. In 2013 we have released a JS-based hyper-local search mobile and web application called Kuwait finder. Today we have more than 650,000 users with an average monthly of 120 thousand active users. These users are generating more than 4 million search operations monthly.
In March 2018 we have released a country-level street view for all of Kuwait and we are still working to enhance and provide better services the State of Kuwait is executing a huge infrastructure project all over the country.
This raises a challenge for us how can we update our Jas data according to these changes. Kuwait finder is generating more than 300 million anonymous GPS logs monthly. Hence the idea came to us how can we utilize the GPS point generated from Kuwait finder to identify our priority areas working with.
These massive data require the use of the proper tools in order to handle visualize and analyze. We used a geo-analytics server to handle this.
Visualizing such massive data of monthly 300 million GPS records would be a struggle the only way to visualize such massive data is to aggregate it into a way that we can actually understand because like now even for just one hour it looks like noise and it’s hard to extract any information from it.
You can inquire about your civil id (PACI) status here.
How To Accomplish PACI?
we are using a geo-analytics server it’s extending the standard tools on objects Enterprise to perform and accelerate the analysis of such spatial-temporal data sets from days to minutes.
So for those logs, we can aggregate them on the neighborhood’s level and we have multiple actually attributes that we can summarize. We start with the users who would like to understand how many users are in each neighborhood another parameter is actually the average speed.
What is the average speed for each neighborhood by running PACI?
it will come back to us in minutes telling us a far better visualization of this massive spatial-temporal data set. This representation is telling us which neighborhood has more activity in terms of the number of users and average speed bottlenecks relative to each other.
But this is not enough. We need to understand the hidden patterns and data structure or structure inside our data we used insights to analyze and explore these patterns.
We can see the impact of one attribute in relation to the others in order to be able to understand any hidden patterns we correlated. With different attributes that we have we use these correlations to create scores and schema in order to prioritize our data based on these scoring systems to acquire PACI.
What are the criteria of PACI?
We created a criteria-based priority list which is given on two levels. The first top five counts and used neighborhood list the second top-five counts and used neighborhood lists at the end.
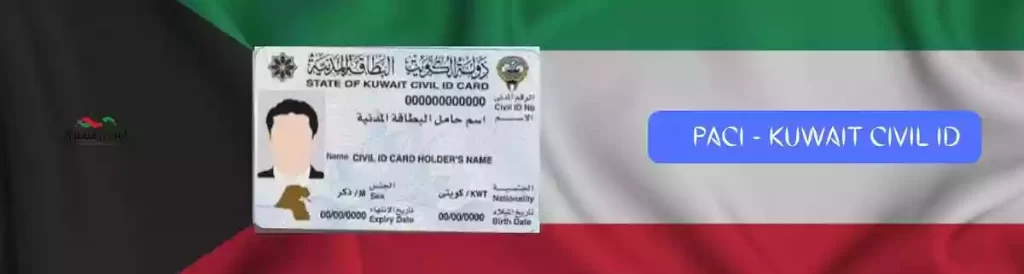
The outcome provides informed decisions rather than random biased and personalized decisions having the output that we got from insight needs to be validated. We need to apply deeper analytics in order to be sure that the result that we are getting is correct.
We used our GIS Pro Analytics to validate our data will be displayed for us using Argo’s pro model builders helped us in combining both off geo-analytics tools and standard tools side-by-side to build such a model which helps in identifying which are the areas that we need to focus on based on the progression over time.
It starts by creating a space-time cube on the right side those space-time cubes are of 1-kilometer precision so for each of both areas we can understand and see the pattern of how it’s evolving over time and which are the hotspots in one of them.
So the next whiz with this is actually to go to the next level which is calculating and emerging hotspots based on all the various attributes that we are having this output helped us actually in pressurizing the areas that we really need to focus on because they have actually a pattern change over there is in few monsters showing activity in terms of a number of users and behavior change of speed and timings are as well.
From this we come up with the top developed areas this is of one-kilometer precision but how this is compared to the outcome coming of the neighborhoods from insights.
We can easily overlay them on top of each other to understand are matching and we can see there is an overlap this tells us that we have now a more granular more accurate representation of the areas that we need to browse and focus our job on using the Geo analytics and the side of the standard tool by side inside the problem.
Thanks to it, our areas are identified but we are starting the mission as we know updating GIS data is time-consuming and laborious what a task and idea came to us how we can use machine learning to automate this process.
What are the outcomes of PACI?
Different approaches were implemented for PACI.
- The first one was applying algorithm-based machine learning.
- we used machine learning to extract street segments from the displaced; none snapped GPS points on the streets and get the extracted streets using elastic principle graphs and self-organizing maps.
- In the second approach that we have applied, we decided to use deep learning and computer vision to extract the street outlines from our newly captured satellite imagery in order to update our data.
Conclusion
This work is still evolving comparing it with reality shows very promising results data-driven decisions are our way for efficiency and productivity for you.
More quality content about Kuwait? Click here